Leveraging the Power of Data Science: Enhancing Your Marketing Strategy
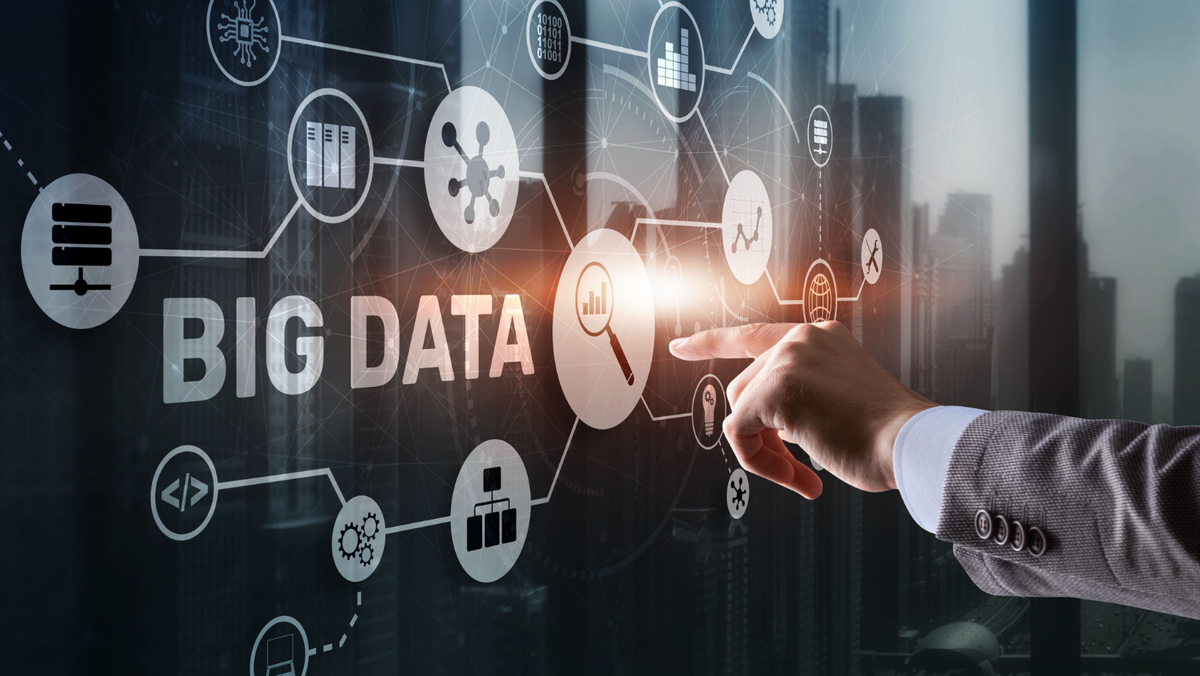
Have you ever noticed that after listening to a song on Spotify, it automatically queues up similar tracks and genres? Or perhaps you've looked up running shoes online, only to find your Instagram flooded with ads from various athletic wear brands like Asics, New Balance, Nike, and Salomon?
These are examples of how companies leveraged data science in marketing!
A successful marketing strategy requires a deep understanding of the customer's wants, needs, and behavior. Data science facilitates through various tools and techniques to collect, analyze, and interpret customer behavior.
Most of us interact with marketing data science applications and subconsciously make decisions based on them.
In this article, I'll discuss several methods through which data science can be utilized in marketing efforts, as well as highlight some excellent platforms/courses to learn more about data science and mastering the role of a marketing data scientist.
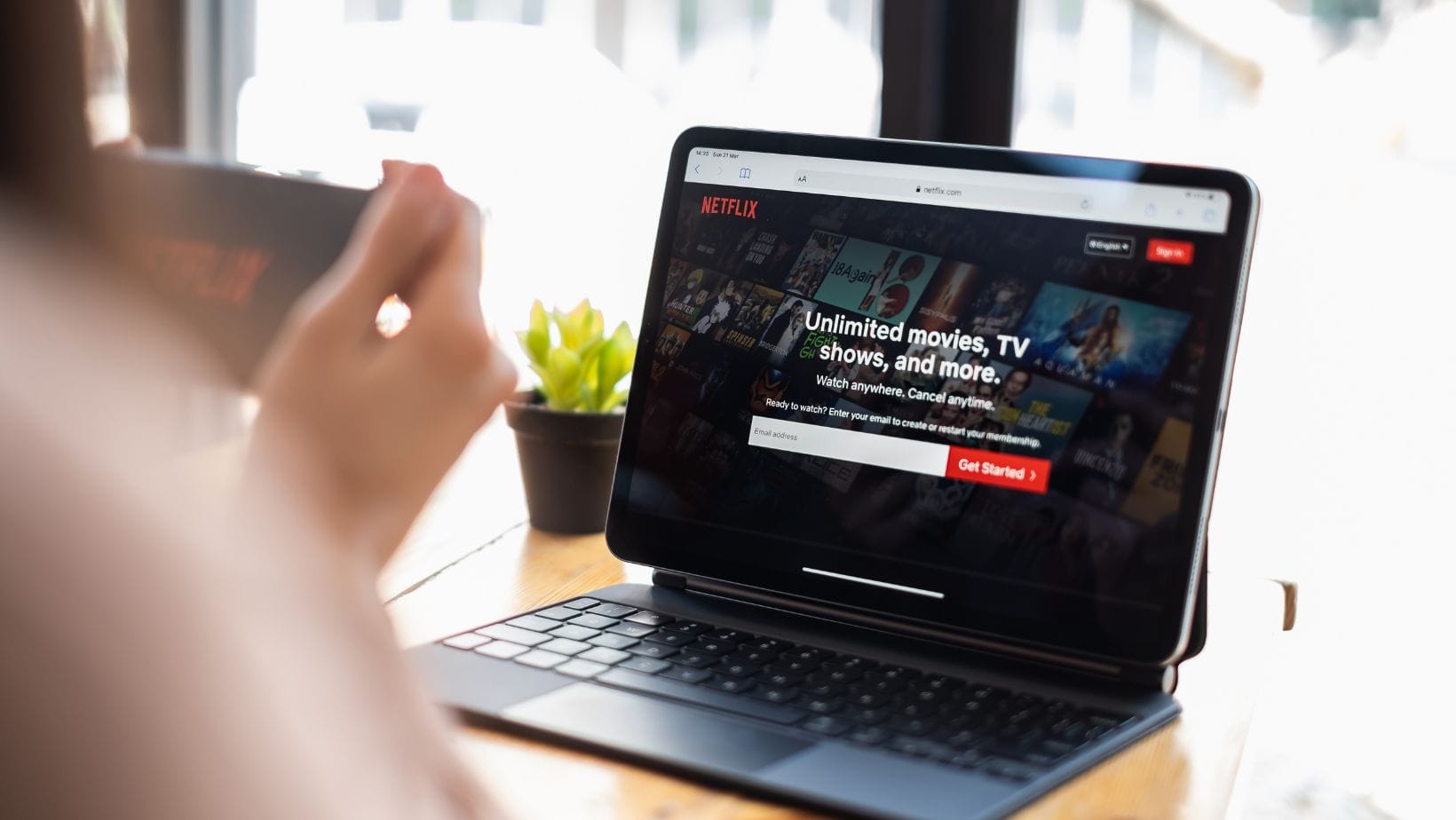
Recommendation Systems
Recommendation models are popular among subscription-based service providers such as Netflix, Spotify, Hulu, and more. The process starts by recommending generic shows and products based on average interest levels and starts getting more and more precise based on your activity on the website. Taking Netflix as an example, the site runs an analysis on the types of shows watched, and the amount of time spent on a specific show, or genre while it creates a custom algorithm that is specific to you.
While it's impressive that this feature suggests shows aligned with your preferences, it comes with the drawback of potentially overlooking new recommendations. For instance, if you enjoy the Harry Potter series and repeatedly watch those movies, the system learns your affinity for supernatural, fantasy, and fictional genres. However, it might miss suggesting non-fiction and suspense genres that you could also enjoy.
To address this limitation, an alternative data science technique comes into play. It involves comparing your viewing habits with those of similar users and then offering recommendations based on these comparisons. This method is known as Collaborative Filtering Based Recommendation Systems, which is just one example of the various types of recommendation systems available.
Churn Prediction
Marketers who leverage data science in their marketing efforts can tell how likely you are to stop using their services even before you realize it or act on it. This is a very powerful tool used for customer retention, called customer churn prediction.
Now imagine that you subscribe to a service, opting for a monthly plan, but then decide to cancel your membership shortly afterward to avoid paying the recurring fee, despite receiving numerous marketing communications and offers just before you unsubscribe.
The challenge here is that by this stage, you've already made up your mind to terminate the service, making it challenging to persuade you to reconsider.
Now imagine a company sending out its promotions and offers mid-month when you’re still on the fence about terminating the service, this makes it way easier to convince you to continue the service with the added benefits.
To take it one step further, data scientists can find out exactly what led to you wanting to terminate your membership and promptly address them by offering solutions, thus ensuring the continuation of your business relationship with the company.
The above example is very straightforward but it captures exactly how useful a predictive model can be leveraged towards customer retention.
Clustering
Clustering essentially uses machine learning and statistics to identify patterns based on behavior, interest, and preferences and divides them into clusters. The first question that comes into mind is, how is that different from customer segmentation?
The difference lies at the core of clustering which is done without any pre-defined segments and is more exploratory in nature. Clustering comes under the unsupervised machine learning model, where an unlabeled data set is used to run an algorithm, leading to an unknown outcome.
The goal is to identify patterns without any intervention to create more accurate customer segments, true to the nature of the dataset.
As we can see in the above image, the dataset is divided into three clusters, ensuring minimum difference individually and maximum difference between clusters.
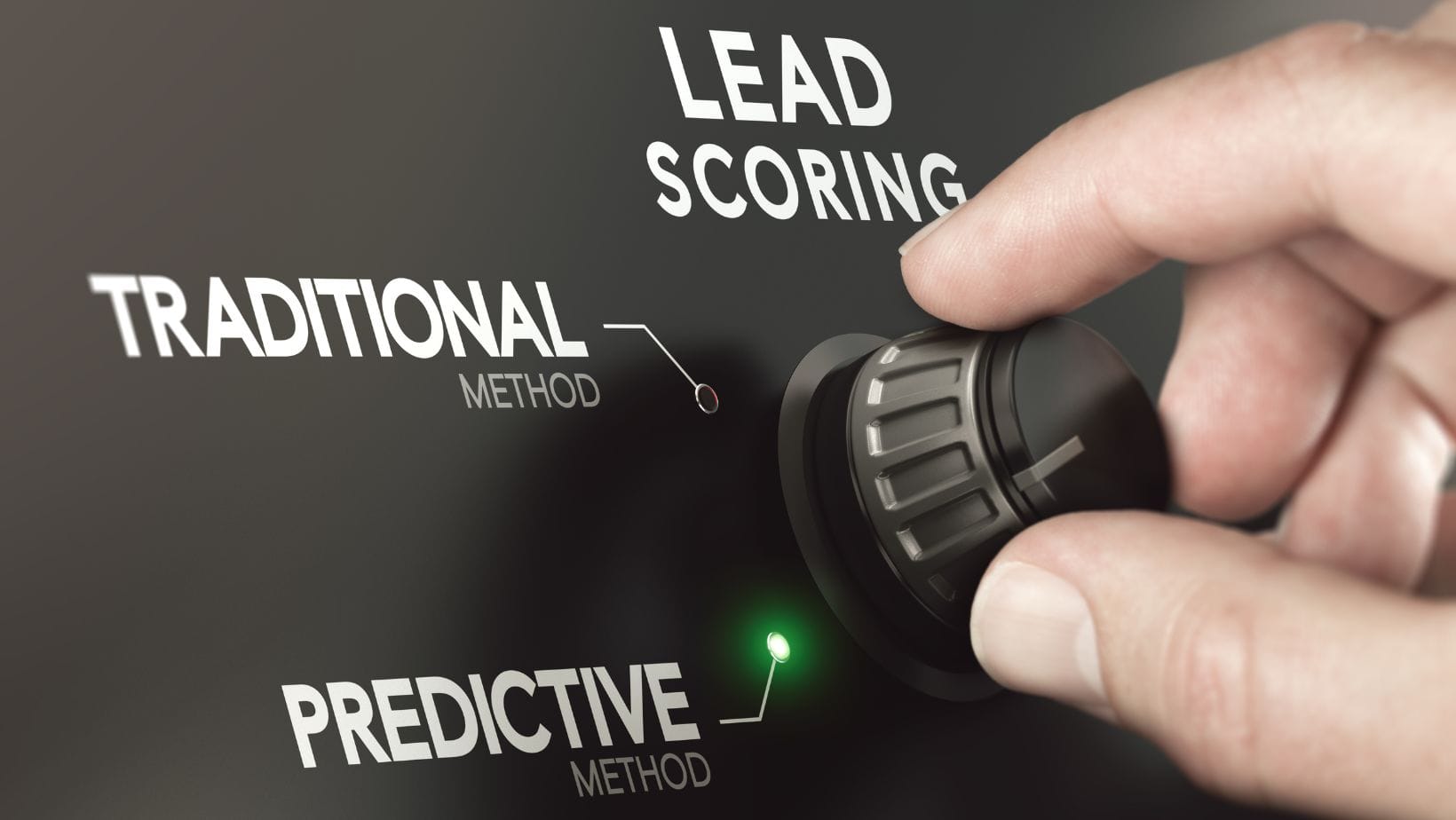
Lead Scoring
This is one of the more common techniques where users are rewarded scores based on their interactions with the website, marketing material, and ads, such as time spent on the website, number of pages viewed, number of emails opened, links clicked, items added to cart, and more.
The model assigns a value to each potential touchpoint, and when engaged, a numerical score is added to the contact information along with its trigger. This allows marketers to promote and curate their marketing efforts to users who are at different stages of the funnel and creates a more conscious marketing strategy.
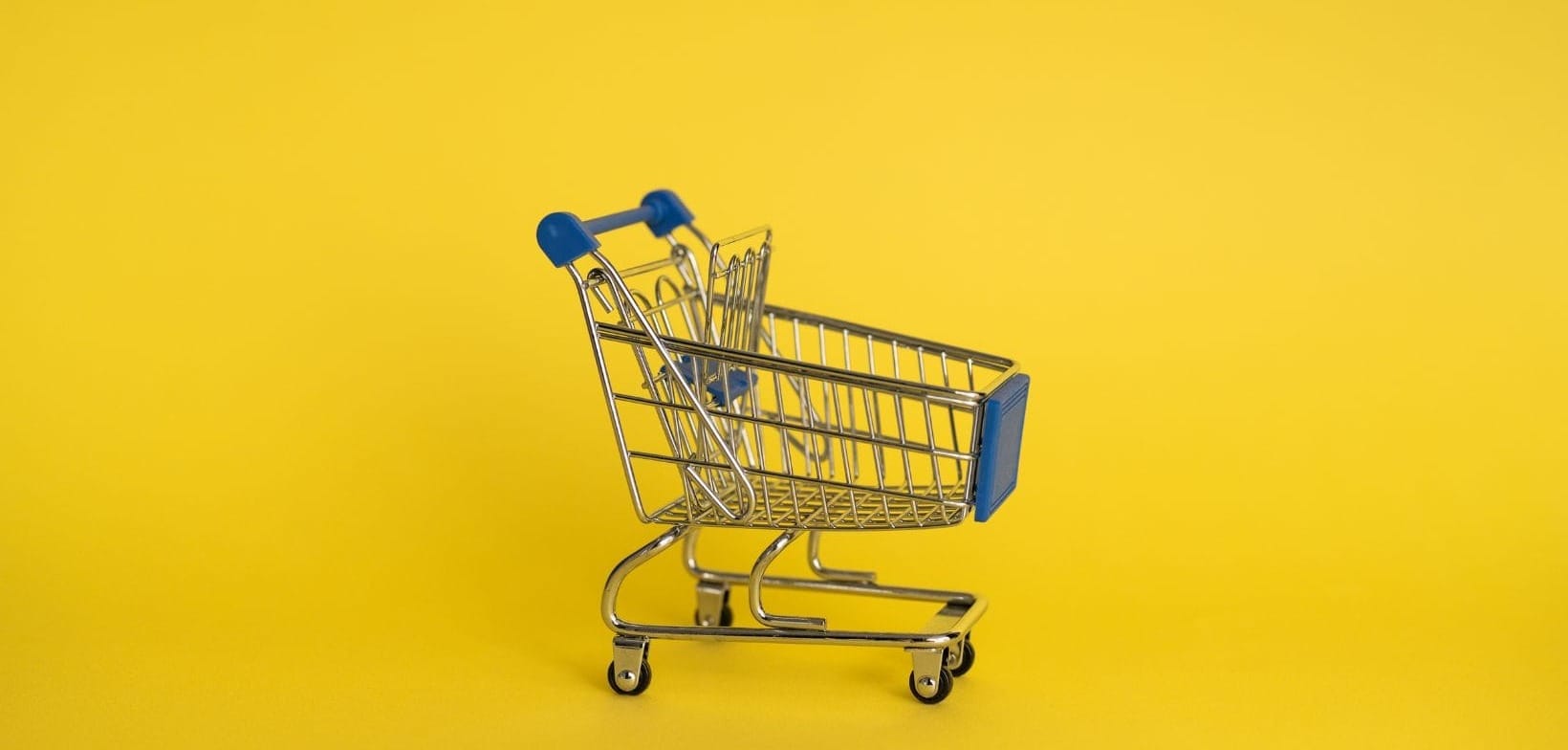
Market Basket Analysis
Market Basket Analysis essentially is the analysis of products frequently brought together to understand the relationship between products and how they can be upsold or grouped. This is often seen in retail stores and restaurants, where for example, baking powder is kept close to flour, to encourage and aid customers to pick both of them up.
Market basket analysis is leveraged by companies to uncover such potential relationships/patterns between products that aren't visible to us, to allow companies and marketers to promote these products better.
There are many use cases for a market basket analysis such as coming up with pricing strategies, showing website content, upselling or grouping products that aren't being sold as much individually, and more.
Regression Analysis
A Regressive Analysis is a technique used when you want to analyze the relationship and impact of an independent variable and a dependent variable, to measure how changes in the independent variable can impact the dependent variable. For example, changes in marketing techniques impact sales.
Regression analysis is a 6 stage process comprising of,
- Defining variables
- Data Collection
- Regression model building
- Analysis
- Interpretation
- Decision-making
The goal is to use this data-driven insight to help formulate pricing, strategies, promotions, and marketing spending.
Affinity Learning
Affinity learning is simply tools using an algorithm to search for frequent item sets and their relationship with each other. This is done via machine learning algorithms such as Apriori, FP-Growth, or the Eclat.
The idea is to leverage the association and relationship of different items to come up with recommendations, product assortment, and marketing promotions.
If this sounds familiar, you're likely thinking of a market basket analysis function, but the key difference is that Affinity learning is item-specific, behavior-specific, and considers the interrelation among them all, whereas market-basket analysis is solely based on purchasing behavior.
Now that we've discussed how data science benefits marketing, you might be excited to leverage these amazing tools and techniques to kickstart your journey as a data scientist.
Before you begin, it is important to understand what makes a good marketing data scientist, and to help you with just that, I have included some must-have hard skills and also highlighted some excellent platforms/courses to facilitate your learning process.
The key skill for excelling as a marketing data scientist is to have strong technical and analytical expertise. Such as:
- A certificate or degree in Statistics, covering various supervised and unsupervised machine learning techniques.
- Proficiency in at least one programming language like SQL, Python, or R.
- Ability to visualize data and create dashboards using tools such as Excel and Tableau.
- Experience conducting A/B tests.
- In-depth understanding of platforms like Facebook and Google ads, including optimization techniques.
These are just a few necessary hard skills required to become a data scientist marketer. It is also important to point out that these requirements may vary from one organization to another.
Now that we've covered what you need to know, here are some recommended courses to help take the first step:
In conclusion, data science has become an indispensable tool for enhancing marketing strategy in today's digital age. By leveraging the power of data analytics, businesses can gain valuable insights, personalize marketing efforts, optimize performance, and stay ahead of the competition. Embracing data science is not just a trend – it's a strategy imperative for businesses looking to thrive in an increasingly competitive marketplace.
As always, Stay tuned and subscribe to stay updated with the ever-changing landscape of Marketing!
Feel free to leave a comment about any questions or possible topics you'd like me to go over!